Maximizing Agriculture Innovation with High-Quality agriculture dataset for machine learning
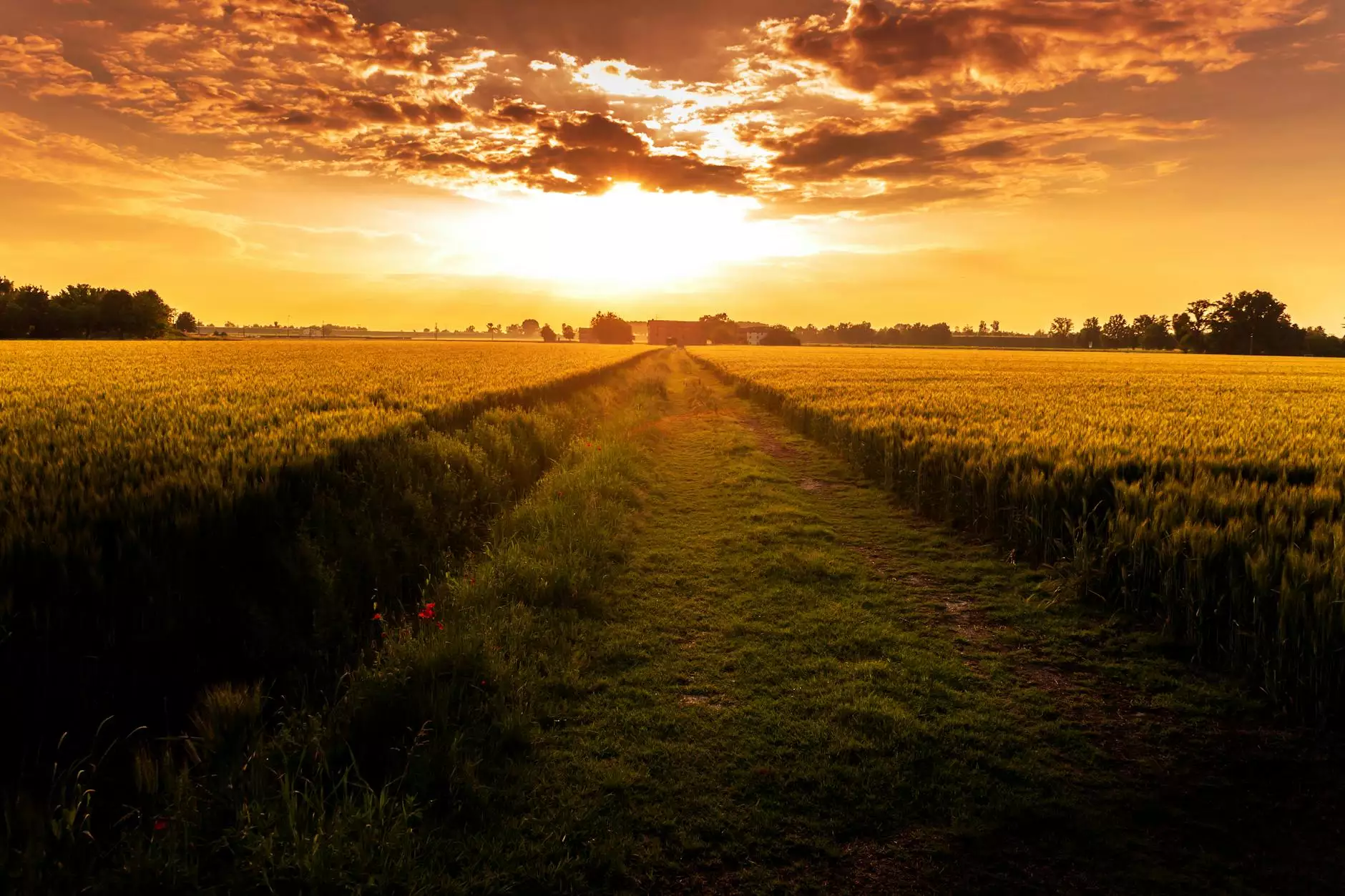
The intersection of agriculture and cutting-edge technology is transforming the landscape of modern farming. Central to this technological revolution is the utilization of agriculture dataset for machine learning, which empowers farmers, researchers, and agribusinesses to optimize crop production, manage resources efficiently, and predict future agricultural trends with unprecedented accuracy. As the industry shifts towards data-driven decision-making, having access to robust, comprehensive datasets becomes not just advantageous but essential for pioneering advancements in software development within agriculture.
Understanding the Role of Agriculture Datasets in Machine Learning
At its core, a agriculture dataset for machine learning is a meticulously collected, organized compilation of relevant agricultural data points. These datasets typically encompass various types of information, including weather patterns, soil quality, crop health images, pest infestations, irrigation schedules, and crop yield data. When fed into machine learning models, this data enables predictive analytics, automated decision-making, and pattern recognition that human analysis alone cannot achieve efficiently.
Importance of High-Quality Agriculture Datasets for Software Development
In the realm of software development, the quality of data directly correlates with the effectiveness and accuracy of the algorithms built. A high-quality agriculture dataset for machine learning possesses several critical attributes:
- Completeness: Covers all necessary parameters such as soil types, crop varieties, climatic conditions, and pest occurrences.
- Accuracy: Data must be precise, validated, and free from errors to ensure reliable model training.
- Consistency: Uniform data collection methods minimize discrepancies and support seamless model integration.
- Timeliness: Up-to-date data enables real-time decision-making and predictive modeling.
- Relevance: The dataset should align with specific agricultural applications, whether crop prediction, pest control, or irrigation management.
Transforming Agriculture with Machine Learning Powered by Datasets
The advent of machine learning in agriculture hinges upon the availability of comprehensive datasets. These datasets serve as the foundation for developing sophisticated models with applications including:
1. Precision Agriculture
Precision agriculture utilizes agriculture datasets for machine learning to optimize inputs such as fertilizers, water, and pesticides. By analyzing soil data, weather patterns, and crop health, AI-driven systems can recommend precise resource application, leading to increased yields, cost savings, and environmental conservation.
2. Crop Yield Prediction
Accurate crop yield prediction enables farmers to plan harvests and market strategies effectively. ML models trained on datasets containing historical yields, weather data, and soil conditions can forecast future harvest quantities with remarkable accuracy.
3. Pest and Disease Detection
Early detection of pests and diseases is crucial to preventing significant crop damage. Image datasets combined with machine learning algorithms allow for real-time identification of infestations, enabling prompt intervention and reducing crop loss.
4. Irrigation and Water Management
Efficient water use is vital in sustainable farming. Datasets capturing soil moisture levels, weather forecasts, and crop water requirements facilitate the development of models that optimize irrigation schedules, conserving water resources while maintaining crop health.
The Critical Role of Data Collection and Dataset Curation
Building a reliable agriculture dataset for machine learning involves rigorous data collection protocols and curation processes. This includes deploying IoT sensors in fields, satellite imagery analysis, drone technology for high-resolution imaging, and manual data recording by agronomists. Ensuring data diversity and representativeness is essential to develop adaptable models that perform well across varied geographic and climatic zones.
Implementation of Datasets in Software Solutions
Once properly curated, these datasets are integrated into software solutions that farm managers, researchers, and policymakers rely on daily. These solutions encompass mobile apps, web-based dashboards, and embedded farm management systems that translate raw data into actionable insights. Specifically, the integration process involves:
- Data preprocessing: Cleaning, normalizing, and transforming data for compatibility.
- Model training: Developing machine learning algorithms using labeled datasets.
- Validation and testing: Ensuring model accuracy and robustness across diverse scenarios.
- Deployment: Integrating models into user-friendly platforms for real-world application.
Benefits of Accessing and Using Agriculture Datasets for Machine Learning
Leveraging high-quality datasets yields numerous benefits:
- Enhanced Productivity: Data-driven decisions lead to optimized resource use and increased crop yields.
- Sustainable Farming: Models promote environmentally friendly practices, reducing chemical runoff and water waste.
- Risk Mitigation: Accurate predictions minimize vulnerabilities to pests, weather extremes, and market fluctuations.
- Cost Reduction: Efficient input management reduces operational expenses.
- Innovation Acceleration: Rich datasets foster the development of novel AI tools and software applications in agriculture.
Challenges and Future Trends in Agriculture Datasets for Machine Learning
Despite the clear advantages, creating and utilizing agriculture datasets pose challenges such as data privacy concerns, varying data standards, and the need for continuous updates. Overcoming these hurdles involves establishing open data collaborations, standardizing data collection protocols, and investing in scalable storage solutions.
Looking ahead, emerging trends include the integration of big data analytics, remote sensing, and Internet of Things (IoT) devices to build more comprehensive datasets. These advancements will enable the deployment of highly adaptive AI models capable of addressing region-specific challenges, ultimately driving a new era of smart agriculture.
Partnering with Keymakr for Exceptional Agriculture Datasets and Software Solutions
As a leader in software development within the agricultural technology space, Keymakr specializes in providing premium agriculture datasets for machine learning. The company offers tailored data collection, annotation, and management services that ensure your datasets are accurate, diverse, and ready for high-performance AI model training. Collaborating with Keymakr means accessing industry expertise and cutting-edge technology to accelerate your agricultural innovation projects.
Conclusion: Unlocking Agricultural Potential with Quality Datasets
The future of agriculture lies in harnessing the power of agriculture dataset for machine learning. High-quality datasets form the backbone of effective AI-powered solutions, enabling smarter farming practices that are productive, sustainable, and resilient. By investing in robust data collection, curation, and integration, stakeholders can unlock new levels of efficiency and technological excellence. Partnering with experts like Keymakr ensures your journey into intelligent agriculture is supported by the best datasets and innovative software development strategies, paving the way for a more sustainable farming future.