The Power of Malware Machine Learning in Modern IT Services
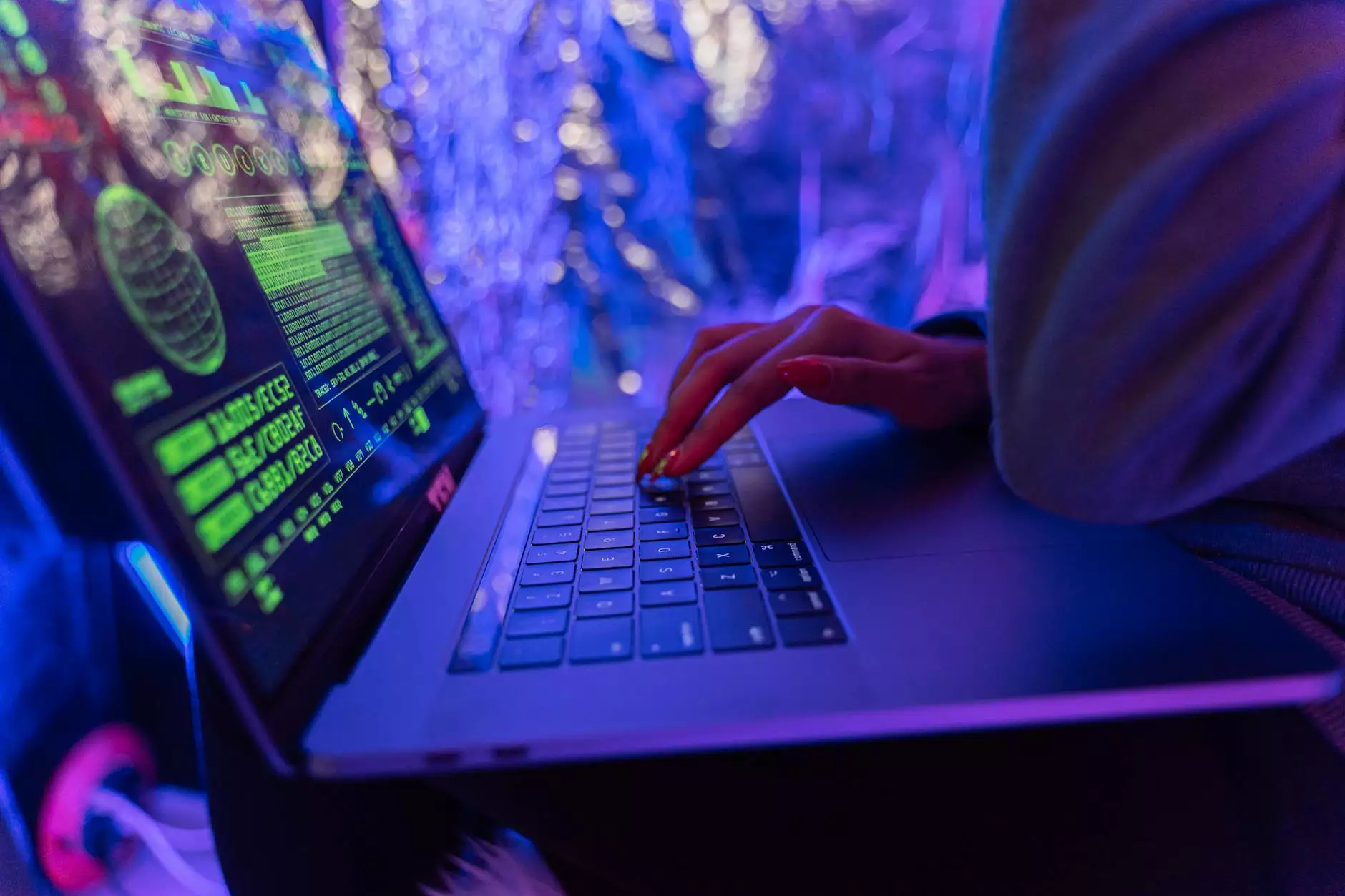
In today's digital landscape, businesses are more vulnerable to cyber threats than ever before. With the rise of sophisticated malware, organizations must leverage innovative technologies to protect their valuable assets. One such advancement that is reshaping the way companies combat malware is machine learning. This article delves into the realm of malware machine learning and how it is elevating IT services and computer repair, along with security systems, to unprecedented levels of efficiency and effectiveness.
What is Malware Machine Learning?
Malware machine learning refers to the integration of machine learning algorithms into the detection and analysis of malicious software. By utilizing large datasets to train predictive models, these algorithms can identify patterns associated with malware, enabling pro-active measures against threats.
The essence of this technology lies in its ability to adapt and evolve as it encounters new types of malware, ensuring that businesses are always one step ahead of cybercriminals. With traditional signature-based detection methods becoming increasingly inadequate against advanced threats, the integration of machine learning presents a crucial evolution in cybersecurity.
How Malware Machine Learning Enhances IT Services
The incorporation of malware machine learning into IT services, particularly at businesses like Spambrella, provides numerous benefits that can bolster an organization's security posture. Let’s explore these benefits in detail:
1. Improved Threat Detection
One of the most significant advantages of machine learning is its capability to learn continuously. By analyzing vast amounts of data, machine learning algorithms can detect anomalous behavior indicative of malware activity. This process involves:
- Behavioral Analysis: Machine learning can monitor user behavior and identify deviations from the norm, such as unusual access patterns or data exfiltration attempts.
- Anomaly Detection: By recognizing what constitutes normal operational behavior, any anomalies can trigger alerts, allowing IT professionals to investigate potential threats promptly.
- Real-Time Threat Analysis: Continuous learning and adaptation mean that threats can be detected in real-time, allowing for immediate response, thereby reducing dwell time for attackers.
2. Automated Responses to Malware Threats
In the fast-paced world of cybersecurity, time is of the essence. Through malware machine learning, IT systems can autonomously respond to detected threats, minimizing the need for human intervention in the preliminary stages of an attack. Automated responses can include:
- Isolation of Infected Systems: Upon detection of a malware outbreak, affected systems can be isolated from the network to prevent lateral movement.
- Automated Threat Mitigation: Actionable insights can be generated to neutralize malware, such as deleting the malware strains or uninstalling infected applications.
- System Recovery: Automated recovery processes can restore affected systems to their last known good configuration, speeding up recovery times significantly.
3. Enhanced Incident Response with Predictive Analytics
Predictive analytics using machine learning can forecast future attacks based on historical data. This foresight allows IT teams to implement preventive measures before an attack happens. The processes involved include:
- Forecasting Attacks: By analyzing past attack vectors, machine learning models can predict where future threats might arise.
- Vulnerability Assessment: Machine learning can aid in identifying weaknesses within the IT infrastructure that could be exploited by cybercriminals.
- Prioritization of Threats: Not all threats are equal; machine learning allows for the prioritization of threats based on potential impact, enabling IT teams to focus on the most critical issues first.
Challenges in Implementing Malware Machine Learning
Despite the numerous advantages offered by malware machine learning, organizations may face several challenges during implementation. These challenges include:
1. Data Quality and Quantity
For machine learning algorithms to perform effectively, they require access to high-quality, diverse datasets. Datasets must consist of various malware types as well as benign software to avoid false positives. Organizations need to invest in collecting and curating comprehensive datasets that reflect the current landscape of cyber threats.
2. The Need for Expertise
Implementing machine learning solutions necessitates a skilled workforce. IT teams must possess expertise not only in cybersecurity but also in machine learning principles and algorithms. This may require continuous training and recruitment of specialized professionals who understand both fields intricately.
3. Cost Implications
The upfront investment in machine learning technology and the ongoing expenses associated with maintaining such systems can be significant. Organizations should perform a cost-benefit analysis to determine the potential return on investment through enhanced security.
Case Studies: Success Stories of Malware Machine Learning in Action
Numerous organizations have successfully implemented malware machine learning to enhance their cybersecurity strategies. Here are a few case studies that exemplify the impact of this technology:
1. Company A: Retail Sector
Company A, a major retailer, encountered a spike in transaction fraud linked to malware infections. By utilizing machine learning for detecting anomalous transaction patterns, they were able to reduce fraudulent transactions by 60% in just six months. The system learned from previous attack vectors, adapting to new methods used by cybercriminals.
2. Company B: Financial Services
A leading financial institution employed a machine learning solution that analyzed network traffic for signs of malware communication with external servers. This proactive measure empowered the company to detect and neutralize 85% of malware threats before any data was exfiltrated.
3. Company C: Healthcare
Company C, a healthcare provider, faced compliance issues due to malware incidents leading to data breaches. By integrating a machine learning-based security system that monitored patient data access patterns, they achieved not only compliance but also fortified their overall cybersecurity framework, reducing malware infection rates by over 70%.
Conclusion: The Future of Malware Machine Learning in Business
As malware becomes increasingly sophisticated, traditional cybersecurity methods will no longer suffice. The future of IT services and computer repair, as well as security systems, heavily relies on the capabilities of malware machine learning. Organizations like Spambrella are at the forefront of embracing these technologies to protect sensitive information and maintain operational integrity.
By investing in machine learning-based solutions, businesses can not only improve their security posture but also ensure business continuity in an increasingly volatile cyber landscape. As we advance into a future dominated by digital transformations, the effective application of malware machine learning will be essential for staying competitive and secure.